Bienvenue au
Laboratoire de cognition et neurodynamique computationnelle
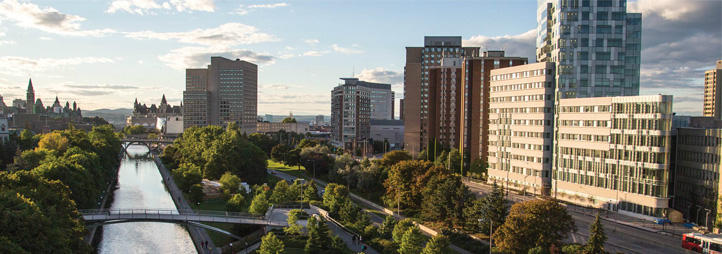
Même si c’est quelque chose que nous faisons tous les jours sans efforts, la mémorisation est en fait une tâche extrêmement complexe. Par exemple, le simple fait d’emmagasiner et extraire des patrons perceptuels est un acte que les ordinateurs ne peuvent accomplir avec l’efficacité et la robustesse de l’humain.
Le laboratoire CONEC vise à mieux comprendre comment le système cognitif humain accompli la tâche complexe de créer (et améliorer) des représentations des patrons tout en étant en mesure de les reconnaitre, identifier, catégoriser et classifier. En particulier, la recherche se concentre sur l’utilisation d’une perspective dynamique nonlinéaire où le temps et le changement sont des variables clés.
Pour comprendre comment le système cognitif humain fonctionne, nous devons développer des modèles formels. Ainsi, le laboratoire CONEC utilise des réseaux de neurones artificiels récurrents qui sont massivement parallèle où l’information est distribuée à l’ensemble des unités. Par conséquent, l’objectif principal est le développement d’une mémoire associative bidirectionnelle qui peuvent rendre compte des apprentissages supervisés, non supervisés et par renforcement tout en étant contraint par les données de la neuroscience. En développant des modèles, nous espérons avoir une meilleure compréhension du fonctionnement du cerveau.
.jpg)
Directeur
Chartier, Sylvain

Directeur du Laboratoire de cognition et neurodynamique computationnelle et Professeurs agrégé, École de psychologie
Pièce : VNR 3022
Courriel professionnel : Sylvain.Chartier@uOttawa.ca
Dr. Sylvain Chartier a obtenu un B.A. de l’université d’Ottawa en 1993, un B.Sc. (honours) de l’université du Québec à Montréal en 1993 et un Ph.D. également de l’université du Québec à Montréal en 2004; tous en psychologie. Sa thèse de doctorat portait sur le développement de réseaux de neurones artificiels pour la catégorisation autonome. De 2004 à 2007, il a effectué un post doctorat au centre de recherche de l’Institut Philippe-Pinel de Montréal où il a effectué des recherches sur les analyses des mouvements oculaires et leur classification. Depuis 2007 il est professeur à l’université d’Ottawa.
Mots-clés
- Mémoires associatives récurrentes
- Systèmes dynamiques nonlinéaires
- Méthodes quantitatives
Contact
Courriel: Sylvain.Chartier@uOttawa.ca
Local: VNR 3022
Chercheur affilié
Cyr, André

Dr André Cyr a gradué en Médecine à l’université de Montréal et a complété un doctorat en Informatique Cognitive à l’Université du Québec à Montréal. Son intérêt premier réside dans la compréhension globale du phénomène de l’intelligence et des liens possibles avec le domaine de l’intelligence artificielle. L’apprentissage et la mémoire sont les thèmes majeurs de ses recherches, étudiés par des approches computationnelles bio-inspirées. La principale méthodologie utilisée est celle des réseaux de neurones artificiels à impulsion (RNAI) agissant comme cerveau-contrôleur d’agents cognitifs complets virtuels et physiques. Les sujets d’étude sont variés mais ont en commun de simuler des caractéristiques ou comportements de bas niveau de l’intelligence naturelle, tel que retrouvés chez les invertébrés. Les différentes hypothèses de recherche sont d’abord explorées par des scénarios virtuels, puis les RNAI sont incarnés dans des robots physiques pour tester les contraintes du monde réel. Les données produites sont expliquées et détaillées du niveau des synapses jusqu’aux comportements des agents cognitifs. Deux exemples actuels de projets sont représentés par la simulation de l’attention visuelle et de l’apprentissage de concepts abstraits tel que semblable/différent.
Mots-Clés
- Intelligence artificielle générale
- Cognition
- Adaptation comportementale
- Mémoire et apprentissage
- Bio-inspiration robotiques
- Neurocience computationnelle
Étudiants du programme d'études supérieures
Berberian, Nareg

B.Sc. Psychologie (2015)
Mots-Clés
- Analysis of electrophysiological data (Multi-electrode Utah Array; Single cell recordings; Calcium imaging; EEG)
- Plasticity in networks of spiking neurons
- Bio-inspired vision and learning in robotics
- Associative memory
- Decision-making
Courriel
Church, Kinsey

Intérêts de recherche: Réseaux de neurones artificiels, cognition, apprentissage, comportement et intelligence artificielles. Mon projet actuel porte sur l’étude du compromis entre l’exploration et l’exploitation en cognition.
Courriel: kchur026@uOttawa.ca
Rolon-Mérette, Damiem

Diplômes: B.Sc. spécialisé en Psychologie et B.Sc. spécialisé approfondie Majeur Biochimie avec Majeur en Psychologie (Année)
Intérêts de recherche : Je me penche présentement sur les mécanismes responsables de l’apprentissage et la mémoire chez l’être humain, plus spécifiquement ceux reliés à l’apprentissage par association. Pour ce faire des réseaux de neurones artificiels sont utilisés afin de modéliser le phénomène. Ceci permet de tirer des conclusions parallèles avec le cerveau humain. Les concepts clés présentement à mon agenda sont les associations de type un-à-multiple, le rôle du contexte dans l’apprentissage par association et comment le tout permettrait d’atteindre de l’intelligence artificielle général.
Email: drolo083@uOttawa.ca
Rolon-Mérette, Thaddé

Depuis 2017, étudiant au doctorat en psychologie expérimentale à l’université d’Ottawa
Reçut un B.Sc.en sciences biomédicales à Université d’Ottawa en 2015
Reçut un B.Sc. en psychologie à Université d’Ottawa en 2016
Ses principaux intérêts de recherche sont les domaines de cognition et réseaux de neurones artificiels, avec une emphase sure:
- Les mémoires associatives
- Apprentissage
- Contextes
- Architecture croissante
- Extraction de caractéristiques
- Apprentissages profond (deep learning)
Ross, Matthew

Étudiants du programme de spécialisation
There are no results for this content list.
Anciens
Laurence Morissette, PhD 2018
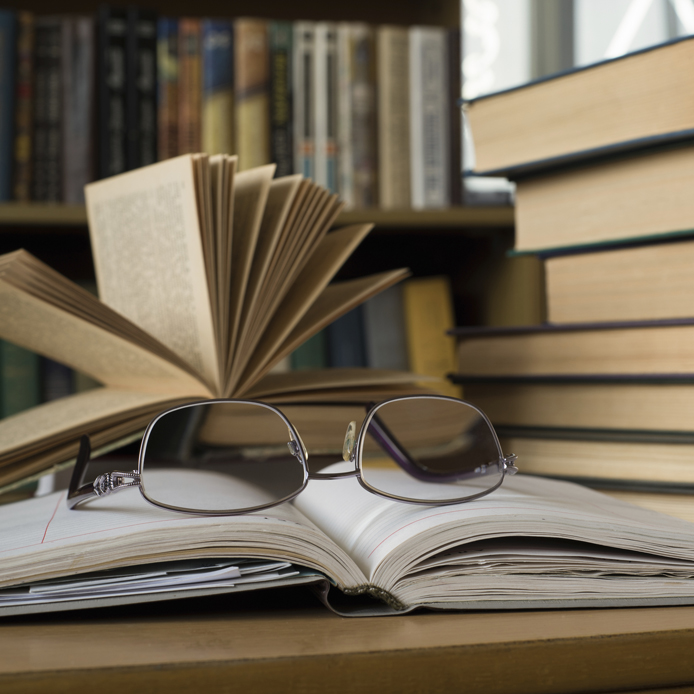
Applications
B
-
Berberian, N., MacPherson, A., Giraud, E., Richardson, L., & Thivierge, J.P. (2017). Neuronal Pattern Separation of Motion-Relevant Input in LIP activity. Journal of Neurophysiology, Vol. 117, No. 2, pp. 738-755.
C
-
Calderini, M., Zhang S., Berberian N., Thivierge J.P., (2018). Optimal Readout of Correlated Neural Activity in a Decision Making Circuit. Neural Computation, Vol. 30, No. 6, pp. 1573-1611.
-
Chartier, S. & Johnson, M. (2015, July). Learning Valid Categorical Syllogisms using an Associative Memory. Proceedings of the International Joint Conference on Neural Networks, Killarney, Ireland, 6 pages.
-
Chartier, S. & Lepage, R. (2002, August). Learning and Extracting Edges from Images by a Modified Hopfield Neural Network. Proceeding of the 16th International Conference on Pattern Recognition, Vol.3, Quebec City, Canada, pp. 431-434.
-
Chartier, S. & Renaud, P. (2006, May). Eye-tracker Data Filtering Using Pulse Coupled Neural Network. Proceeding of the 17th IASTED International Conference on Modelling and Simulation, Montréal, Canada, pp. 91-96.
-
Chartier, S. & Renaud, P. (2008, March). An Online Noise Filter for Eye-Tracker Data Recorded in a Virtual Environment. Proceedings of Eye Tracking Research & Applications Symposium, Savannah, USA, pp. 153-156.
-
Chartier, S., Cousineau, D., & Charbonneau, D. (2004, July). A Connexionist Model of the Attentional Blink Effect During a Rapid Serial Visual Presentation Task. In M. Lovett, C. Schunn, C. Lebiere, P. Munro (Eds.) Proceedings of the Sixth International Conference on Cognitive Modelling, (pp. 64-69). Mahwah, NJ: Lawrence Erlbaum Associates Publishers. Pittsburgh, USA.
-
Chartier, S., Leth-Steensen, C. & Hébert, M.-F. (2012). Performing Complex Associations Using a Generalized Bidirectional Associative Memory. Journal of Experimental & Theoretical Artificial Intelligence, Vol. 24, No. 1, pp. 23-42.
-
Chartier, S., Renaud, P. & Caron, S. (2007, June). Autonomous Classification of Sexual Offenders from Eye Pattern Behavior, In G. Bourgon, R.K. Hanson, J.D. Pozzulo, K.E. Morton Bourgon, & C.L. Tanasichuk (Eds.), The Proceedings of the 2007 North American Correctional & Criminal Justice Psychology Conference (pp. 158-161). Ottawa: Public Safety Canada.
-
Chartier, S., Renaud, P., Bouchard, S., Proulx, J., Rouleau, J. L., Fedoroff P., & Bradford, J., (2006). Sexual Preference Classification from Gaze Behavior Data using a Multilayer Perceptron, Annual Review of CyberTherapy and Telemedicine, vol. 4, pp. 149-157.
-
Cyr, A., & Thériault, F. (2015). Action Selection and Operant Conditioning: A Neurorobotic Implementation. Journal of Robotics. Vol. 2015, Article ID 643869, 10 pages.
H
-
Hébert, M.-F., Chartier, S., & Tremblay, C. (2014, July). Solving Valid Syllogistic Problems using a Bidirectional Heteroassociative Memory. Proceedings of the 36th Annual Meeting of the Cognitive Science Society, Quebec City, Canada, pp. 2345-2350.
-
Hélie, S., Chartier, S. & Proulx, R. (2006). Are Unsupervised Neural Networks Ignorant? Sizing the Effect of the Environmental Distribution on Unsupervised Learning. Cognitive Systems Research, vol. 7, no. 4, pp. 357-371.
L
-
Lahmiri, S., Boukadoum, M. & Chartier, S. (2010, May). Daily Stock Market Forecasting using Volume: A comparison of ANFIS and Time Delay Recurrent Neural Networks. Sixth International Conference on Intelligent Systems: Theory and Applications. Rabat, Morocco, 10 pages.
-
Lahmiri, S. Boukadoum, M. & Chartier, S. (2013, May) Information Fusion and S&P500 Trend Prediction. Proceedings of the International Conference on Computer Systems and Applications, FES, Morocco. 6 pages.
-
Lahmiri S., Boukadoum, M. & Chartier, S. (2013). A supervised classification system of financial data based on wavelet packet and neural networks. International Journal of Strategic Decision Sciences, Vol. 4, No. 4, pp. 72-84.
-
Lahmiri S., Boukadoum, M. & Chartier, S. (2014). Exploring Information Categories and Artificial Neural Networks Numerical Algorithms in S&P500 Trend Prediction: A Comparative Study. International Journal of Strategic Decision Sciences, vol. 5, no. 1, pages 76-94.
-
Leth-Steensen, C., Chartier, S., Langlois, D. & Hébert, M. (2010, May). Performing Complex Associations using a Feature-Extracting Bidirectional Associative Memory. In H. W. Guesgen & R. C. Murray (Eds.), Florida Artificial Intelligence Research Society Conference (pp. 367-372). Florida, USA: AAAI Press.
M
-
Morissette, L. & Chartier, S. (2015, July). Saliency model of auditory attention based on frequency, amplitude and spatial location. Proceedings of the International Joint Conference on Neural Networks, Killarney, Ireland, 6 pages.
-
Morissette, L., Chartier, S., Vandermeulen, R. & Watier, N. (2012). Depth of Treatment Sensitive Noise Resistant Dynamic Artificial Neural Networks Model of Recall in People with Prosopagnosia. Neural Networks, Vol. 32, pp.46-56.
N
-
Nejadgholi, I., Seyyedsalehi, S. A. & Chartier, S. (2012). Chaotic Feature Extracting BAM and Its Application in Implementing Memory Search. Neural Processing Letters, Vol. 36, pp. 69-99.
O
-
Orbán, L. & Chartier, S. (2013, April). Unsupervised non-linear neural networks capture aspects of floral choice behavior. Proceedings of the European Symposium on Artificial Neural Networks, Computational Intelligence and Machine Learning, Bruges, Belgium, pp. 149-154.
-
Orbán, L. L., Plowright CMS, Chartier, S., Thompson, E., & Xu, V. (2015) Visual Choice Behaviour by Bumblebees (Bombus impatiens) Confirms Unsupervised Neural Network’s Prediction. Journal of Comparative Psychology. Vol.129, No. 3, 229-236.
R
-
Ross, M., Payeur, P. & Chartier, S. (2019, June). Task Allocation for Heterogeneous Robots Using a Self-Organizing Contextual Map. IEEE International Symposium on RObotic and Sensors Environments (ROSE), Ottawa, Canada. 6 pages.
T
-
Tabari, K., Boukadoum, M., Chartier, S. & Lounis. H. (2006, December) Reconnaissance d’expressions faciales à l’aide d’une mémoire associative bidirectionnelle à fonction de sortie chaotique. Proceedings of Maghrebian Conference on Software Engineering and Artificial Intelligence, Agadir, Morocco, pp. 422-426.
-
Tremblay, C., Harding, B., Chartier, S., & Cousineau, D. (2014). System Factorial Technology applied to Artificial Neural Network Information Processing. In B. Goertzel, L. Orseau, J. Snaider (Eds.) Artificial General Intelligence: Lecture Notes in Computer Science, (pp. 258-261). Springer International Publishing.
V
-
Vandermeulen, R., Morrissette, L. & Chartier, S. (2011, July). Modeling Prosopagnosia Using Dynamic Artificial Neural Networks. Proceedings of the International Joint Conference on Neural Networks, San Jose, USA. pp. 2074-2079.
W
-
Wu, W., Payeur, P., Al-Buraiki, O., & Ross, M. (2019, August). Vision-Based Target Objects Recognition and Segmentation for Unmanned Systems Task Allocation. Proceedings of the International Conference on Image Analysis and Recognition, Waterloo, Canada, pp. 252-263.
Extraction de composantes
C
-
Chartier, S. & Giguère, G. (2008, July). Autonomous Perceptual Feature Extraction in a Topology-Constrained Architecture. In B. C. Love, K. McRae, & V. M. Sloutsky (Eds.), Proceedings of the 30th Annual Conference of the Cognitive Science Society (pp. 1868-1873). Austin, TX: Cognitive Science Society.
-
Chartier, S., Giguère, G., & Langlois, D. (2009). A new bidirectional heteroassociative memory encompassing correlational, competitive and topological properties. Neural Networks, Vol. 22, No. 5-6, pp. 568-578.
-
Chartier, S., Giguère, G., Langlois, D & Sioufi, R. (2009, June) Bidirectional Associative Memories, Self-Organizing Maps and k-Winners-Take-All: Uniting Feature Extraction and Topological Principles, Proceedings of the International Joint Conference on Neural Networks, Atlanta, USA, pp. 503-510.
-
Chartier, S., Giguère, G., Renaud, P., Lina, J.-M., & Proulx, R. (2007, August). FEBAM: A Feature-Extracting Bidirectional Associative Memory, Proceedings of the International Joint Conference on Neural Networks. Orlando, USA, pp. 1679-1684.
-
Chartier, S., Leth-Steensen, C. & Hébert, M.-F. (2012). Performing Complex Associations Using a Generalized Bidirectional Associative Memory. Journal of Experimental & Theoretical Artificial Intelligence, Vol. 24, No. 1, pp. 23-42.
G
-
Giguère, G., Chartier, S., Proulx, R., & Lina, J.-M. (2007, July). Creating Perceptual Features Using a BAM Architecture, In D. S. McNamara & J. G. Trafton (Eds.), Proceedings of the 29th Annual Cognitive Science Society (pp. 1025-1030). Austin, TX: Cognitive Science Society.
-
Giguère, G., Chartier, S., Proulx, R., Lina, J.-M. (2007, July). Category development and reorganization using a bidirectional associative memory-inspired architecture. In R.L. Lewis, T.A. Polk, & J.E. Laird (Eds.), Proceedings of the 8th International Conference on Cognitive Modeling, (pp. 97-102). Ann Arbor, Mi: University of Michigan.
L
-
Leth-Steensen, C., Chartier, S., Langlois, D. & Hébert, M. (2010, May). Performing Complex Associations using a Feature-Extracting Bidirectional Associative Memory. In H. W. Guesgen & R. C. Murray (Eds.), Florida Artificial Intelligence Research Society Conference (pp. 367-372). Florida, USA: AAAI Press.
M
-
Morissette, L., Chartier, S., Vandermeulen, R. & Watier, N. (2012). Depth of Treatment Sensitive Noise Resistant Dynamic Artificial Neural Networks Model of Recall in People with Prosopagnosia. Neural Networks, Vol. 32, pp.46-56.
-
Morrissette, L. & Chartier, S. (2013, August). FEBAMSOM-BAM*: Neural Network Model of Human Categorization of the N-Bits Parity Problem. Proceedings of the International Joint Conference on Neural Networks, Dallas, USA, pp. 1897-1901.
N
-
Nejadgholi, I., Chartier, S. & Seyyedsalehi, S. A. (2013) Controlling Deterministic Output Variability in a Feature Extracting Chaotic BAM. Neurocomputing, Vol. 120, pp. 298-309.
-
Nejadgholi, I., SeyyedSalehi, S. A. & Chartier, S. (2017). A Brain-Inspired Method of Facial Expression Generation Using Chaotic Feature Extracting Bidirectional Associative Memory. Neural Processing Letters. Vol. 46, No. 3, pp 943–960.
-
Nejadgholi, I., Seyyedsalehi, S. A. & Chartier, S. (2012). Chaotic Feature Extracting BAM and Its Application in Implementing Memory Search. Neural Processing Letters, Vol. 36, pp. 69-99.
O
-
Orbán, L. & Chartier, S. (2013, April). Unsupervised non-linear neural networks capture aspects of floral choice behavior. Proceedings of the European Symposium on Artificial Neural Networks, Computational Intelligence and Machine Learning, Bruges, Belgium, pp. 149-154.
-
Orbán, L.L., & Chartier, S. (2015). Unsupervised neural network quantifies the cost of visual information processing. PLoS One. Vol.10, No. 7, e0132218, 14 pages.
R
-
Rolon-Merette, T., Rolon-Merette, D. & Chartier, S. (2018). Generating Cognitive Context with Feature-Extracting Bidirectional Associative Memory. Procedia Computer Science, vol. 145, pp.428-436.
-
Rolon-Mérette, T., Rolon-Mérette, D. & Chartier, S. (2019, July). Different Brain, Same Prototype? Cognitive Variability within a Recurrent Associative Memory. Proceedings of the International Conference on Cognitive Modeling, Montréal, Canada. 6 pages.
-
Ross, M., Chartier, S., & Hélie, S. (2017). The neurodynamics of categorization: Critical challenges and proposed solutions. In Claire Lefebvre & Henri Cohen (Eds.), Handbook of Categorization in Cognitive Science, (1053-1076). Elsevier.
T
-
Tremblay, C., Myers-Stewart, K., Morissette, L., & Chartier, S. (2013, July). Bidirectional Associative Memory and Learning of Nonlinearly Separable Tasks. In R. West & T. Stewart (Eds.), Proceedings of the 12th International Conference on Cognitive Modeling, Ottawa, Canada, pp. 420-425.
V
-
Vandermeulen, R., Morrissette, L. & Chartier, S. (2011, July). Modeling Prosopagnosia Using Dynamic Artificial Neural Networks. Proceedings of the International Joint Conference on Neural Networks, San Jose, USA. pp. 2074-2079.
Mémoire
B
-
Berberian, N., Aamir, Z., Hélie, S. & Chartier, S. (2016, July). Encoding Sparse Features in a Bidirectional Associative Memory. IEEE World Congress on Computational Intelligence, Vancouver, Canada, pp. 5119-5126.
C
-
Chartier, S. & Boukadoum, M. (2006). A New Bidirectional Heteroassociative Memory for Binary and Grey-Level Patterns. IEEE Transactions on Neural Networks, vol. 17, no. 2, pp. 385 - 396.
-
Chartier, S. & Boukadoum, M. (2006). A Sequential Dynamic Heteroassociative Memory for MultiStep Pattern Recognition and One-to-Many Association. IEEE Transactions on Neural Networks, vol. 17, no. 1, pp. 59-68.
-
Chartier, S. & Boukadoum, M. (2006, December) A Chaotic Bidirectional Associative Memory. Proceedings of Maghrebian Conference on Software Engineering and Artificial Intelligence, Agadir, Morocco, pp. 498-501.
-
Chartier, S. & Boukadoum, M. (2011). Encoding Static and Temporal Patterns with a Bidirectional Heteroassociative Memory. Journal of Applied Mathematics. Article ID 301204, 31 pages.
-
Chartier, S. & Giguère, G. (2008, July). Autonomous Perceptual Feature Extraction in a Topology-Constrained Architecture. In B. C. Love, K. McRae, & V. M. Sloutsky (Eds.), Proceedings of the 30th Annual Conference of the Cognitive Science Society (pp. 1868-1873). Austin, TX: Cognitive Science Society.
-
Chartier, S. & Lepage, R. (2002, August). Learning and Extracting Edges from Images by a Modified Hopfield Neural Network. Proceeding of the 16th International Conference on Pattern Recognition, Vol.3, Quebec City, Canada, pp. 431-434.
-
Chartier, S. & Proulx, R. (1999, July). A Self-Scaling Procedure in Unsupervised Correlational Neural Networks, Proceedings of the International Joint Conference on Neural Networks, Vol. 2, Washington D.C., USA, pp. 1092-1096.
-
Chartier, S. & Proulx, R. (2001, July). A New Online Unsupervised Learning Rule for the BSB Model, Proceedings of the International Joint Conference on Neural Networks, Vol. 1, Washington D.C., USA, pp. 448-453.
-
Chartier, S. & Proulx, R. (2005). NDRAM: A Nonlinear Dynamic Recurrent Associative Memory for Learning Bipolar and Nonbipolar Correlated Patterns. IEEE Transactions on Neural Networks, vol. 16, no. 6, pp. 1393-1400.
-
Chartier, S., Giguère, G., & Langlois, D. (2009). A new bidirectional heteroassociative memory encompassing correlational, competitive and topological properties. Neural Networks, Vol. 22, No. 5-6, pp. 568-578.
-
Chartier, S., Giguère, G., Langlois, D & Sioufi, R. (2009, June) Bidirectional Associative Memories, Self-Organizing Maps and k-Winners-Take-All: Uniting Feature Extraction and Topological Principles, Proceedings of the International Joint Conference on Neural Networks, Atlanta, USA, pp. 503-510.
-
Chartier, S., Giguère, G., Renaud, P., Lina, J.-M., & Proulx, R. (2007, August). FEBAM: A Feature-Extracting Bidirectional Associative Memory, Proceedings of the International Joint Conference on Neural Networks. Orlando, USA, pp. 1679-1684.
-
Chartier, S., Hélie, S., Boukadoum, M. & Proulx, R. (2005, August). SCRAM: Statistically Converging Recurrent Associative Memory. Proceedings of the International Joint Conference on Neural Networks, 2, Montréal, Canada, pp. 723-728.
-
Chartier S., Renaud, P & Boukadoum, M. (2008). A Nonlinear Dynamic Artificial Neural Network Model of Memory, New Ideas in Psychology, vol. 26, no 2, pp. 252-277.
-
Cyr A., Thériault F., Ross M., Chartier S. (2018, August) Associative Memory: An Spiking Neural Network Robotic Implementation. In Iklé M., Franz A., Rzepka R., Goertzel B. (eds) Artificial General Intelligence 2018. Lecture Notes in Computer Science, (31-41). Springer
G
-
Giguère, G., Chartier, S., Proulx, R., & Lina, J.-M. (2007, July). Creating Perceptual Features Using a BAM Architecture, In D. S. McNamara & J. G. Trafton (Eds.), Proceedings of the 29th Annual Cognitive Science Society (pp. 1025-1030). Austin, TX: Cognitive Science Society.
-
Giguère, G., Chartier, S., Proulx, R., Lina, J.-M. (2007, July). Category development and reorganization using a bidirectional associative memory-inspired architecture. In R.L. Lewis, T.A. Polk, & J.E. Laird (Eds.), Proceedings of the 8th International Conference on Cognitive Modeling, (pp. 97-102). Ann Arbor, Mi: University of Michigan.
H
-
Harding, B., Goulet, M.-A., Cousineau, D. & Chartier, S. (2017, May). Are Recurrent Associative Memories Good Models of Decision Making? Modelling discrimination decisions from different perspectives Bradley Harding, Proceedings of the International Joint Conference on Neural Networks, Anchorage, USA, pages 2621-2628.
J
-
Jeanson, F., & Chartier, S. (2013, July). Memory Control in a FitzHugh-Nagumo Network via STDP. In R. West & T. Stewart (Eds.). Proceedings of the 12th International Conference on Cognitive Modeling, Ottawa, Canada, pp. 137-142.
-
Johnson, M. & Chartier, S. (2018, July). Is There a Purpose to Network Redundancy? Proceedings of the International Joint Conference on Neural Networks, Rio de Janeiro, Brasil. 8 pages.
-
Johnson, M., & Chartier, S. (2014). Increasing Accuracy in a Bidirectional Associative Memory through Expended Databases. In B. Goertzel, L. Orseau, J. Snaider (Eds.) Artificial General Intelligence: Lecture Notes in Computer Science, (pp. 53-62). Springer International Publishing.
-
Johnson, M., & Chartier, S. (2017, September). Model Derived Spike Time Dependent Plasticity. In Lintas A., Rovetta S., Verschure P., Villa A. (eds). Artificial Neural Networks and Machine Learning – ICANN 2017, Alghero, Italy, pages 345-353.
M
-
Morissette, L., Chartier, S., Vandermeulen, R. & Watier, N. (2012). Depth of Treatment Sensitive Noise Resistant Dynamic Artificial Neural Networks Model of Recall in People with Prosopagnosia. Neural Networks, Vol. 32, pp.46-56.
N
-
Nejadgholi, I., SeyyedSalehi, S. A. & Chartier, S. (2017). A Brain-Inspired Method of Facial Expression Generation Using Chaotic Feature Extracting Bidirectional Associative Memory. Neural Processing Letters. Vol. 46, No. 3, pp 943–960.
R
-
Rolon-Mérette, D., Rolon-Mérette, T. & Chartier, S. (2018, July). Distinguishing Highly Correlated Patterns using a Context Based Approach in Bidirectional Associative Memory. Proceedings of the International Joint Conference on Neural Networks, Rio de Janeiro, Brasil. 8 pages
-
Rolon-Mérette, D., Rolon-Mérette, T. & Chartier, S. (2019, July). Learning and Recalling Arbitrary Lists of Overlapping Exemplars in a Recurrent Artificial Neural Network. Proceedings of the International Conference on Cognitive Modeling, Montréal, Canada. 6 pages.
-
Rolon-Merette, T., Rolon-Merette, D. & Chartier, S. (2018). Generating Cognitive Context with Feature-Extracting Bidirectional Associative Memory. Procedia Computer Science, vol. 145, pp.428-436.
-
Rolon-Mérette, T., Rolon-Mérette, D. & Chartier, S. (2019, July). Different Brain, Same Prototype? Cognitive Variability within a Recurrent Associative Memory. Proceedings of the International Conference on Cognitive Modeling, Montréal, Canada. 6 pages.
-
Ross, M., Chartier, S., & Hélie, S. (2017). The neurodynamics of categorization: Critical challenges and proposed solutions. In Claire Lefebvre & Henri Cohen (Eds.), Handbook of Categorization in Cognitive Science, (1053-1076). Elsevier.
S
-
Salmon R., Sadeghian A. & Chartier, S. (2010, July). Reinforcement Learning using Associative Memory Networks. Proceedings of the International Joint Conference on Neural Networks, Barcelona, Spain, 7 pages.
T
-
Tremblay, C. & Chartier, S. (2014, May). BAM Learning in High Level of Connection Sparseness. Proceedings of the Twenty-Seventh International Florida Artificial Intelligence Research Society Conference, Pensacola Beach, USA, pp. 97-100.
-
Tremblay, C., Berberian, N., & Chartier, S., (2014, July). A new Bidirectional Associative Memory for Short-term Memory Learning. Proceedings of the 36th Annual Meeting of the Cognitive Science Society, Quebec City, Canada, pp. 1917-1922.
Z
-
Zamani, M., Sadeghian A. & Chartier, S. (2010, July). A Bidirectional Associative Memory Based on Cortical Spiking Neurons Using Temporal Coding. Proceedings of the International Joint Conference on Neural Networks, Barcelona, Spain, 7 pages.
Réseaux de neurones à impulsions
B
-
Berberian, N., MacPherson, A., Giraud, E., Richardson, L., & Thivierge, J.P. (2017). Neuronal Pattern Separation of Motion-Relevant Input in LIP activity. Journal of Neurophysiology, Vol. 117, No. 2, pp. 738-755.
-
Berberian, N., Ross, M. & Chartier, S. (2019). Discrimination of Motion Direction in a Robot Using a Phenomenological Model of Synaptic Plasticity. Computational Intelligence and Neuroscience. Article ID 6989128, 14 pages.
-
Berberian, N., Ross, M., Chartier, S., Thievierge, J.-P. (2017, December). Synergy Between Short-Term and Long-Term Plasticity Explains Direction-Selectivity in Visual Cortex. IEEE Symposium Series on Computational Intelligence (SSCI), Honolulu, USA. 8 pages.
C
-
Calderini, M., Zhang S., Berberian N., Thivierge J.P., (2018). Optimal Readout of Correlated Neural Activity in a Decision Making Circuit. Neural Computation, Vol. 30, No. 6, pp. 1573-1611.
-
Chartier, S. & Renaud, P. (2006, May). Eye-tracker Data Filtering Using Pulse Coupled Neural Network. Proceeding of the 17th IASTED International Conference on Modelling and Simulation, Montréal, Canada, pp. 91-96.
-
Cyr, A., & Boukadoum, M. (2012). Classical conditioning in different temporal constraints: an STDP learning rule for robots controlled by spiking neural networks. Adaptive Behavior, Vol. 20, No 4, pp. 257-272.
-
Cyr, A., & Boukadoum, M. (2013). Habituation: a non-associative learning rule design for spiking neurons and an autonomous mobile robots implementation. Bioinspiration & Biomimetics, Vol. 8, No.1, 016007.
-
Cyr, A., & Thériault, F. (2015). Action Selection and Operant Conditioning: A Neurorobotic Implementation. Journal of Robotics. Vol. 2015, Article ID 643869, 10 pages.
-
Cyr, A., & Thériault, F. (2019). Spatial Concept Learning: A Spiking Neural Network Implementation in Virtual and Physical Robots. Computational Intelligence and Neuroscience, Article ID 8361369, 8 pages.
-
Cyr, A., Avarguès-Weber, A. & Thériault,F. (2017). Sameness/difference spiking neural circuit as a relational concept precursor model: A bio-inspired robotic implementation,
Biologically Inspired Cognitive Architectures,Vol. 21, pp. 59-66. -
Cyr, A., Boukadoum, M., & Poirier, P. (2009). AI-SIMCOG: a simulator for spiking neurons and multiple animats’ behaviours. Neural Computing and Applications, Vol. 18, No. 5, 431-446.
-
Cyr, A., Boukadoum, M., & Thériault, F. (2012). NeuroSim: A Virtual 3D-World to Investigate the Intelligence Phenomenon within the Perspective of Bio-inspired Robotic Agents. In Virtual worlds: Artificial ecosystems and digital art exploration. Bornhofen, S., et al. eds., Science eBooks, Chapter 12, pp. 167-185.
-
Cyr, A., Boukadoum, M., & Thériault, F. (2014). Operant conditioning: a minimal components requirement in artificial spiking neurons designed for bio-inspired robot's controller. Frontiers in neurorobotics, 8.
-
Cyr, A., Morand-Ferron, J., & Thériault, F. (2020). Dual exploration strategies using artificial spiking neural networks in a robotic learning task. Adaptive Behavior, 1059712320924744.
-
Cyr, A., Theriault, F. (2019): Bio-inspired visual attention process using spiking neural networks controlling a camera. In press. International Journal of Computational Vision and Robotics, vol. 9, no. 1, pp. 39-55.
-
Cyr, A., Thériault, F., & Chartier, S. (2019). Revisiting the XOR problem: a neurorobotic implementation. Neural Computing and Applications, 1-9. DOI:10.1007/s00521-019-04522-0
-
Cyr, A., Thériault, F., Ross, M., Berberian, N. & Chartier, S. (2018). Spiking Neurons Integrating Visual Stimuli Orientation and Direction Selectivity in a Robotic Context. Frontiers in Neurorobotics vol. 12, Article 75, 10 pages.
-
Cyr A., Thériault F., Ross M., Chartier S. (2018, August) Associative Memory: An Spiking Neural Network Robotic Implementation. In Iklé M., Franz A., Rzepka R., Goertzel B. (eds) Artificial General Intelligence 2018. Lecture Notes in Computer Science, (31-41). Springer
J
-
Jeanson, F., & Chartier, S. (2013, July). Memory Control in a FitzHugh-Nagumo Network via STDP. In R. West & T. Stewart (Eds.). Proceedings of the 12th International Conference on Cognitive Modeling, Ottawa, Canada, pp. 137-142.
-
Johnson, M. & Chartier, S. (2018). Spike Neural Models Part II: Abstract Neural Models. Tutorials in Quantitative Methods for Psychology, Vol. 14, No. 1, 2018, pp. 1-16.
-
Johnson, M., & Chartier, S. (2017, September). Model Derived Spike Time Dependent Plasticity. In Lintas A., Rovetta S., Verschure P., Villa A. (eds). Artificial Neural Networks and Machine Learning – ICANN 2017, Alghero, Italy, pages 345-353.
-
Johnson., M. & Chartier, S. (2017). Spike neural models Part I: The Hodgkin-Huxley model, The Quantitative Methods for Psychology, Vol. 13, no. 2, pp. 105-119.
M
-
Morissette, L. & Chartier, S. (2015, July). Saliency model of auditory attention based on frequency, amplitude and spatial location. Proceedings of the International Joint Conference on Neural Networks, Killarney, Ireland, 6 pages.
R
-
Ross, M., Berberian, N., Cyr, A., Thériault, F., Chartier, S. (2017, September). Learning Distance-Behavioural Preferences Using a Single Sensor in a Spiking Neural Network. In Lintas A., Rovetta S., Verschure P., Villa A. (eds). Artificial Neural Networks and Machine Learning – ICANN 2017, Alghero, Italy, pp.110-118.
Z
-
Zamani, M., Sadeghian A. & Chartier, S. (2010, July). A Bidirectional Associative Memory Based on Cortical Spiking Neurons Using Temporal Coding. Proceedings of the International Joint Conference on Neural Networks, Barcelona, Spain, 7 pages.
Réseaux de neurones artificiels
A
-
Amiri, M., Davande, H., Sadeghian, A. & Chartier, S. (2010). Feedback Associative Memory Based on a New Hybrid Model of Generalized Regression and Self Feedback Neural Networks. Neural Networks, Vol. 23, No. 7, 892-904.
-
Amiri, M., Sadeghian, A, & Chartier, S. (2010, July). One-shot Training Algorithm for Self-Feedback Neural Networks. North American Fuzzy Information Processing Society, Toronto, Canada. pp. 104-109.
B
-
Berberian, N., Aamir, Z., Hélie, S. & Chartier, S. (2016, July). Encoding Sparse Features in a Bidirectional Associative Memory. IEEE World Congress on Computational Intelligence, Vancouver, Canada, pp. 5119-5126.
C
-
Chartier, S. & Boukadoum, M. (2006). A New Bidirectional Heteroassociative Memory for Binary and Grey-Level Patterns. IEEE Transactions on Neural Networks, vol. 17, no. 2, pp. 385 - 396.
-
Chartier, S. & Boukadoum, M. (2006). A Sequential Dynamic Heteroassociative Memory for MultiStep Pattern Recognition and One-to-Many Association. IEEE Transactions on Neural Networks, vol. 17, no. 1, pp. 59-68.
-
Chartier, S. & Boukadoum, M. (2006, December) A Chaotic Bidirectional Associative Memory. Proceedings of Maghrebian Conference on Software Engineering and Artificial Intelligence, Agadir, Morocco, pp. 498-501.
-
Chartier, S. & Boukadoum, M. (2011). Encoding Static and Temporal Patterns with a Bidirectional Heteroassociative Memory. Journal of Applied Mathematics. Article ID 301204, 31 pages.
-
Chartier, S. & Giguère, G. (2008, July). Autonomous Perceptual Feature Extraction in a Topology-Constrained Architecture. In B. C. Love, K. McRae, & V. M. Sloutsky (Eds.), Proceedings of the 30th Annual Conference of the Cognitive Science Society (pp. 1868-1873). Austin, TX: Cognitive Science Society.
-
Chartier, S. & Johnson, M. (2015, July). Learning Valid Categorical Syllogisms using an Associative Memory. Proceedings of the International Joint Conference on Neural Networks, Killarney, Ireland, 6 pages.
-
Chartier, S. & Lepage, R. (2002, August). Learning and Extracting Edges from Images by a Modified Hopfield Neural Network. Proceeding of the 16th International Conference on Pattern Recognition, Vol.3, Quebec City, Canada, pp. 431-434.
-
Chartier, S. & Proulx, R. (1999, July). A Self-Scaling Procedure in Unsupervised Correlational Neural Networks, Proceedings of the International Joint Conference on Neural Networks, Vol. 2, Washington D.C., USA, pp. 1092-1096.
-
Chartier, S. & Proulx, R. (2001, July). A New Online Unsupervised Learning Rule for the BSB Model, Proceedings of the International Joint Conference on Neural Networks, Vol. 1, Washington D.C., USA, pp. 448-453.
-
Chartier, S. & Proulx, R. (2005). NDRAM: A Nonlinear Dynamic Recurrent Associative Memory for Learning Bipolar and Nonbipolar Correlated Patterns. IEEE Transactions on Neural Networks, vol. 16, no. 6, pp. 1393-1400.
-
Chartier, S. & Renaud, P. (2008, March). An Online Noise Filter for Eye-Tracker Data Recorded in a Virtual Environment. Proceedings of Eye Tracking Research & Applications Symposium, Savannah, USA, pp. 153-156.
-
Chartier, S., Boukadoum, M. and Amiri, M. (2009), BAM Learning of Nonlinearly Separable Tasks by Using an Asymmetrical Output Function and Reinforcement Learning, IEEE Transactions on Neural Networks, vol. 20, no. 8, pp. 1281-1292.
-
Chartier, S., Cousineau, D., & Charbonneau, D. (2004, July). A Connexionist Model of the Attentional Blink Effect During a Rapid Serial Visual Presentation Task. In M. Lovett, C. Schunn, C. Lebiere, P. Munro (Eds.) Proceedings of the Sixth International Conference on Cognitive Modelling, (pp. 64-69). Mahwah, NJ: Lawrence Erlbaum Associates Publishers. Pittsburgh, USA.
-
Chartier, S., Giguère, G., & Langlois, D. (2009). A new bidirectional heteroassociative memory encompassing correlational, competitive and topological properties. Neural Networks, Vol. 22, No. 5-6, pp. 568-578.
-
Chartier, S., Giguère, G., Langlois, D & Sioufi, R. (2009, June) Bidirectional Associative Memories, Self-Organizing Maps and k-Winners-Take-All: Uniting Feature Extraction and Topological Principles, Proceedings of the International Joint Conference on Neural Networks, Atlanta, USA, pp. 503-510.
-
Chartier, S., Giguère, G., Renaud, P., Lina, J.-M., & Proulx, R. (2007, August). FEBAM: A Feature-Extracting Bidirectional Associative Memory, Proceedings of the International Joint Conference on Neural Networks. Orlando, USA, pp. 1679-1684.
-
Chartier, S., Hélie, S., Boukadoum, M. & Proulx, R. (2005, August). SCRAM: Statistically Converging Recurrent Associative Memory. Proceedings of the International Joint Conference on Neural Networks, 2, Montréal, Canada, pp. 723-728.
-
Chartier, S., Leth-Steensen, C. & Hébert, M.-F. (2012). Performing Complex Associations Using a Generalized Bidirectional Associative Memory. Journal of Experimental & Theoretical Artificial Intelligence, Vol. 24, No. 1, pp. 23-42.
-
Chartier, S., Renaud, P. & Caron, S. (2007, June). Autonomous Classification of Sexual Offenders from Eye Pattern Behavior, In G. Bourgon, R.K. Hanson, J.D. Pozzulo, K.E. Morton Bourgon, & C.L. Tanasichuk (Eds.), The Proceedings of the 2007 North American Correctional & Criminal Justice Psychology Conference (pp. 158-161). Ottawa: Public Safety Canada.
-
Chartier, S., Renaud, P., Bouchard, S., Proulx, J., Rouleau, J. L., Fedoroff P., & Bradford, J., (2006). Sexual Preference Classification from Gaze Behavior Data using a Multilayer Perceptron, Annual Review of CyberTherapy and Telemedicine, vol. 4, pp. 149-157.
-
Chartier S., Renaud, P & Boukadoum, M. (2008). A Nonlinear Dynamic Artificial Neural Network Model of Memory, New Ideas in Psychology, vol. 26, no 2, pp. 252-277.
-
Church, K. A., Ross, M. & Chartier, S. (2020, July). Using a Bidirectional Associative Memory and Feature Extraction to model Nonlinear Exploitation Problems. Problems. Proceedings of the International Conference on Cognitive Modeling, Held online, 7 pages.
D
-
Davande, H., Amiri, M., Sadeghian, A. & Chartier, S., (2008, June) Auto-associative memory based on a new hybrid model of SFNN and GRNN: performance comparison with NDRAM, ART2 and MLP. Proceedings of the International Joint Conference on Neural Networks, Hong Kong, China, pp. 1698-1703.
G
-
Giguère, G., Chartier, S., Proulx, R., & Lina, J.-M. (2007, July). Creating Perceptual Features Using a BAM Architecture, In D. S. McNamara & J. G. Trafton (Eds.), Proceedings of the 29th Annual Cognitive Science Society (pp. 1025-1030). Austin, TX: Cognitive Science Society.
-
Giguère, G., Chartier, S., Proulx, R., Lina, J.-M. (2007, July). Category development and reorganization using a bidirectional associative memory-inspired architecture. In R.L. Lewis, T.A. Polk, & J.E. Laird (Eds.), Proceedings of the 8th International Conference on Cognitive Modeling, (pp. 97-102). Ann Arbor, Mi: University of Michigan.
H
-
Harding, B., Goulet, M.-A., Cousineau, D. & Chartier, S. (2017, May). Are Recurrent Associative Memories Good Models of Decision Making? Modelling discrimination decisions from different perspectives Bradley Harding, Proceedings of the International Joint Conference on Neural Networks, Anchorage, USA, pages 2621-2628.
-
Hébert, M.-F., Chartier, S., & Tremblay, C. (2014, July). Solving Valid Syllogistic Problems using a Bidirectional Heteroassociative Memory. Proceedings of the 36th Annual Meeting of the Cognitive Science Society, Quebec City, Canada, pp. 2345-2350.
-
Hélie, S., Chartier, S. & Proulx, R. (2006). Are Unsupervised Neural Networks Ignorant? Sizing the Effect of the Environmental Distribution on Unsupervised Learning. Cognitive Systems Research, vol. 7, no. 4, pp. 357-371.
J
-
Johnson, M. & Chartier, S. (2018, July). Is There a Purpose to Network Redundancy? Proceedings of the International Joint Conference on Neural Networks, Rio de Janeiro, Brasil. 8 pages.
-
Johnson, M., & Chartier, S. (2014). Increasing Accuracy in a Bidirectional Associative Memory through Expended Databases. In B. Goertzel, L. Orseau, J. Snaider (Eds.) Artificial General Intelligence: Lecture Notes in Computer Science, (pp. 53-62). Springer International Publishing.
-
Johnson, M., & Chartier, S. (2017, September). Model Derived Spike Time Dependent Plasticity. In Lintas A., Rovetta S., Verschure P., Villa A. (eds). Artificial Neural Networks and Machine Learning – ICANN 2017, Alghero, Italy, pages 345-353.
L
-
Lahmiri, S., Boukadoum, M. & Chartier, S. (2010, May). Daily Stock Market Forecasting using Volume: A comparison of ANFIS and Time Delay Recurrent Neural Networks. Sixth International Conference on Intelligent Systems: Theory and Applications. Rabat, Morocco, 10 pages.
-
Lahmiri, S. Boukadoum, M. & Chartier, S. (2013, May) Information Fusion and S&P500 Trend Prediction. Proceedings of the International Conference on Computer Systems and Applications, FES, Morocco. 6 pages.
-
Lahmiri S., Boukadoum, M. & Chartier, S. (2013). A supervised classification system of financial data based on wavelet packet and neural networks. International Journal of Strategic Decision Sciences, Vol. 4, No. 4, pp. 72-84.
-
Lahmiri S., Boukadoum, M. & Chartier, S. (2014). Exploring Information Categories and Artificial Neural Networks Numerical Algorithms in S&P500 Trend Prediction: A Comparative Study. International Journal of Strategic Decision Sciences, vol. 5, no. 1, pages 76-94.
-
Leth-Steensen, C., Chartier, S., Langlois, D. & Hébert, M. (2010, May). Performing Complex Associations using a Feature-Extracting Bidirectional Associative Memory. In H. W. Guesgen & R. C. Murray (Eds.), Florida Artificial Intelligence Research Society Conference (pp. 367-372). Florida, USA: AAAI Press.
M
-
Morissette, L., Chartier, S., Vandermeulen, R. & Watier, N. (2012). Depth of Treatment Sensitive Noise Resistant Dynamic Artificial Neural Networks Model of Recall in People with Prosopagnosia. Neural Networks, Vol. 32, pp.46-56.
-
Morrissette, L. & Chartier, S. (2013, August). FEBAMSOM-BAM*: Neural Network Model of Human Categorization of the N-Bits Parity Problem. Proceedings of the International Joint Conference on Neural Networks, Dallas, USA, pp. 1897-1901.
N
-
Nejadgholi, I., Chartier, S. & Seyyedsalehi, S. A. (2013) Controlling Deterministic Output Variability in a Feature Extracting Chaotic BAM. Neurocomputing, Vol. 120, pp. 298-309.
-
Nejadgholi, I., SeyyedSalehi, S. A. & Chartier, S. (2017). A Brain-Inspired Method of Facial Expression Generation Using Chaotic Feature Extracting Bidirectional Associative Memory. Neural Processing Letters. Vol. 46, No. 3, pp 943–960.
-
Nejadgholi, I., Seyyedsalehi, S. A. & Chartier, S. (2012). Chaotic Feature Extracting BAM and Its Application in Implementing Memory Search. Neural Processing Letters, Vol. 36, pp. 69-99.
O
-
Orbán, L. & Chartier, S. (2013, April). Unsupervised non-linear neural networks capture aspects of floral choice behavior. Proceedings of the European Symposium on Artificial Neural Networks, Computational Intelligence and Machine Learning, Bruges, Belgium, pp. 149-154.
-
Orbán, L.L., & Chartier, S. (2015). Unsupervised neural network quantifies the cost of visual information processing. PLoS One. Vol.10, No. 7, e0132218, 14 pages.
R
-
Rolon-Mérette, D., Rolon-Mérette, T. & Chartier, S. (2018, July). Distinguishing Highly Correlated Patterns using a Context Based Approach in Bidirectional Associative Memory. Proceedings of the International Joint Conference on Neural Networks, Rio de Janeiro, Brasil. 8 pages
-
Rolon-Mérette, D., Rolon-Mérette, T. & Chartier, S. (2019, July). Learning and Recalling Arbitrary Lists of Overlapping Exemplars in a Recurrent Artificial Neural Network. Proceedings of the International Conference on Cognitive Modeling, Montréal, Canada. 6 pages.
-
Rolon-Merette, T., Rolon-Merette, D. & Chartier, S. (2018). Generating Cognitive Context with Feature-Extracting Bidirectional Associative Memory. Procedia Computer Science, vol. 145, pp.428-436.
-
Rolon-Mérette, T., Rolon-Mérette, D. & Chartier, S. (2019, July). Different Brain, Same Prototype? Cognitive Variability within a Recurrent Associative Memory. Proceedings of the International Conference on Cognitive Modeling, Montréal, Canada. 6 pages.
-
Ross, M., Berberian, N. & Chartier, S. (2020, July) Should I Stay or Should I Grow? A Dynamic Self-Governed Growth for Determining Hidden Layer Size in a Multilayer Perceptron. Proceedings of the International Joint Conference on Neural Networks. Held Online, 8 pages.
-
Ross, M., Chartier, S., & Hélie, S. (2017). The neurodynamics of categorization: Critical challenges and proposed solutions. In Claire Lefebvre & Henri Cohen (Eds.), Handbook of Categorization in Cognitive Science, (1053-1076). Elsevier.
-
Ross, M., Payeur, P. & Chartier, S. (2019, June). Task Allocation for Heterogeneous Robots Using a Self-Organizing Contextual Map. IEEE International Symposium on RObotic and Sensors Environments (ROSE), Ottawa, Canada. 6 pages.
S
-
Salmon R., Sadeghian A. & Chartier, S. (2010, July). Reinforcement Learning using Associative Memory Networks. Proceedings of the International Joint Conference on Neural Networks, Barcelona, Spain, 7 pages.
T
-
Tabari, K., Boukadoum, M., Chartier, S. & Lounis. H. (2006, December) Reconnaissance d’expressions faciales à l’aide d’une mémoire associative bidirectionnelle à fonction de sortie chaotique. Proceedings of Maghrebian Conference on Software Engineering and Artificial Intelligence, Agadir, Morocco, pp. 422-426.
-
Tremblay, C. & Chartier, S. (2014, May). BAM Learning in High Level of Connection Sparseness. Proceedings of the Twenty-Seventh International Florida Artificial Intelligence Research Society Conference, Pensacola Beach, USA, pp. 97-100.
-
Tremblay, C., Berberian, N., & Chartier, S., (2014, July). A new Bidirectional Associative Memory for Short-term Memory Learning. Proceedings of the 36th Annual Meeting of the Cognitive Science Society, Quebec City, Canada, pp. 1917-1922.
-
Tremblay, C., Dorville, M., Myers, K. & Chartier, S. (2013, August). Spreading Activation and Sparseness in a Bidirectional Associative Memory. Proceedings of the International Joint Conference on Neural Networks, Dallas, USA, pp. 1917-1923.
-
Tremblay, C., Harding, B., Chartier, S., & Cousineau, D. (2014). System Factorial Technology applied to Artificial Neural Network Information Processing. In B. Goertzel, L. Orseau, J. Snaider (Eds.) Artificial General Intelligence: Lecture Notes in Computer Science, (pp. 258-261). Springer International Publishing.
-
Tremblay, C., Myers-Stewart, K., Morissette, L., & Chartier, S. (2013, July). Bidirectional Associative Memory and Learning of Nonlinearly Separable Tasks. In R. West & T. Stewart (Eds.), Proceedings of the 12th International Conference on Cognitive Modeling, Ottawa, Canada, pp. 420-425.
V
-
Vandermeulen, R., Morrissette, L. & Chartier, S. (2011, July). Modeling Prosopagnosia Using Dynamic Artificial Neural Networks. Proceedings of the International Joint Conference on Neural Networks, San Jose, USA. pp. 2074-2079.
W
-
Wu, W., Payeur, P., Al-Buraiki, O., & Ross, M. (2019, August). Vision-Based Target Objects Recognition and Segmentation for Unmanned Systems Task Allocation. Proceedings of the International Conference on Image Analysis and Recognition, Waterloo, Canada, pp. 252-263.
Z
-
Zamani, M., Sadeghian A. & Chartier, S. (2010, July). A Bidirectional Associative Memory Based on Cortical Spiking Neurons Using Temporal Coding. Proceedings of the International Joint Conference on Neural Networks, Barcelona, Spain, 7 pages.
Robotique
B
-
Berberian, N., Ross, M. & Chartier, S. (2019). Discrimination of Motion Direction in a Robot Using a Phenomenological Model of Synaptic Plasticity. Computational Intelligence and Neuroscience. Article ID 6989128, 14 pages.
C
-
Cyr, A., & Boukadoum, M. (2012). Classical conditioning in different temporal constraints: an STDP learning rule for robots controlled by spiking neural networks. Adaptive Behavior, Vol. 20, No 4, pp. 257-272.
-
Cyr, A., & Boukadoum, M. (2013). Habituation: a non-associative learning rule design for spiking neurons and an autonomous mobile robots implementation. Bioinspiration & Biomimetics, Vol. 8, No.1, 016007.
-
Cyr, A., & Thériault, F. (2015). Action Selection and Operant Conditioning: A Neurorobotic Implementation. Journal of Robotics. Vol. 2015, Article ID 643869, 10 pages.
-
Cyr, A., & Thériault, F. (2019). Spatial Concept Learning: A Spiking Neural Network Implementation in Virtual and Physical Robots. Computational Intelligence and Neuroscience, Article ID 8361369, 8 pages.
-
Cyr, A., Avarguès-Weber, A. & Thériault,F. (2017). Sameness/difference spiking neural circuit as a relational concept precursor model: A bio-inspired robotic implementation,
Biologically Inspired Cognitive Architectures,Vol. 21, pp. 59-66. -
Cyr, A., Boukadoum, M., & Poirier, P. (2009). AI-SIMCOG: a simulator for spiking neurons and multiple animats’ behaviours. Neural Computing and Applications, Vol. 18, No. 5, 431-446.
-
Cyr, A., Boukadoum, M., & Thériault, F. (2012). NeuroSim: A Virtual 3D-World to Investigate the Intelligence Phenomenon within the Perspective of Bio-inspired Robotic Agents. In Virtual worlds: Artificial ecosystems and digital art exploration. Bornhofen, S., et al. eds., Science eBooks, Chapter 12, pp. 167-185.
-
Cyr, A., Boukadoum, M., & Thériault, F. (2014). Operant conditioning: a minimal components requirement in artificial spiking neurons designed for bio-inspired robot's controller. Frontiers in neurorobotics, 8.
-
Cyr, A., Morand-Ferron, J., & Thériault, F. (2020). Dual exploration strategies using artificial spiking neural networks in a robotic learning task. Adaptive Behavior, 1059712320924744.
-
Cyr, A., Theriault, F. (2019): Bio-inspired visual attention process using spiking neural networks controlling a camera. In press. International Journal of Computational Vision and Robotics, vol. 9, no. 1, pp. 39-55.
-
Cyr, A., Thériault, F., & Chartier, S. (2019). Revisiting the XOR problem: a neurorobotic implementation. Neural Computing and Applications, 1-9. DOI:10.1007/s00521-019-04522-0
-
Cyr, A., Thériault, F., Ross, M., Berberian, N. & Chartier, S. (2018). Spiking Neurons Integrating Visual Stimuli Orientation and Direction Selectivity in a Robotic Context. Frontiers in Neurorobotics vol. 12, Article 75, 10 pages.
R
-
Ross, M., Berberian, N., Cyr, A., Thériault, F., Chartier, S. (2017, September). Learning Distance-Behavioural Preferences Using a Single Sensor in a Spiking Neural Network. In Lintas A., Rovetta S., Verschure P., Villa A. (eds). Artificial Neural Networks and Machine Learning – ICANN 2017, Alghero, Italy, pp.110-118.
-
Ross, M., Payeur, P. & Chartier, S. (2019, June). Task Allocation for Heterogeneous Robots Using a Self-Organizing Contextual Map. IEEE International Symposium on RObotic and Sensors Environments (ROSE), Ottawa, Canada. 6 pages.
W
-
Wu, W., Payeur, P., Al-Buraiki, O., & Ross, M. (2019, August). Vision-Based Target Objects Recognition and Segmentation for Unmanned Systems Task Allocation. Proceedings of the International Conference on Image Analysis and Recognition, Waterloo, Canada, pp. 252-263.
Tutoriels
C
-
Chartier, S. & Allaire, J.-F. (2007). Power Estimation in MANOVA. The Quantitative Methods for Psychology, vol. 3, no. 2, pp. 70-78.
-
Chartier, S. & Cousineau, D. (2011). Computing Mixed-Design (split-plot) ANOVA. The Mathematica Journal, Vol. 13, 22 pages.
-
Chartier, S. & Faulkner, A. (2008). General Linear Models: An Integrated Approaches to Statistics. The Quantitative Methods for Psychology, vol. 4, no. 2, pp. 65-78.
-
Cousineau, D. & Chartier, S. (2010) Outliers detection and treatment: A review. International Journal of Psychological Research, Vol. 3, No. 1, 58-67.
J
-
Johnson, M. & Chartier, S. (2018). Spike Neural Models Part II: Abstract Neural Models. Tutorials in Quantitative Methods for Psychology, Vol. 14, No. 1, 2018, pp. 1-16.
-
Johnson., M. & Chartier, S. (2017). Spike neural models Part I: The Hodgkin-Huxley model, The Quantitative Methods for Psychology, Vol. 13, no. 2, pp. 105-119.
L
-
Langlois, D. Chartier, S. & Gosselin, D. (2010). Independent component analysis. The Quantitative Methods for Psychology, Vol. 6, No. 1, 31-38.
M
-
Morissette, L. & Chartier, S. (2013). The k-means clustering technique: general considerations and implementation in Mathematica. The Quantitative Methods for Psychology, Vol. 9, No. 1, pp. 15-24.
V
-
Van Roon, P., Zakizadeh, J., & Chartier, S. (2014). Partial Least Squares tutorial for analyzing neuroimaging data. The Quantitative Methods for Psychology, Vol. 10, No. 2, pages 200-215.
W
-
Watier, N., Lamontagne, C. & Chartier, S. (2011). What does the mean mean? Journal of Statistics Education, Vol. 19, No. 2, 20 pages.
-
Watier, N., Lamontagne, C. & Chartier, S. (2014) Descriptive Statistics. Probability and Statistics. In José I. Barragués, Adolfo Morais & Jenaro Guisasola (Eds.), Probability and Statistics: A Didactic Introduction, (pp. 1-37). CRC Press.
Vision
B
-
Berberian, N., Ross, M. & Chartier, S. (2019). Discrimination of Motion Direction in a Robot Using a Phenomenological Model of Synaptic Plasticity. Computational Intelligence and Neuroscience. Article ID 6989128, 14 pages.
C
-
Cyr, A., Thériault, F., Ross, M., Berberian, N. & Chartier, S. (2018). Spiking Neurons Integrating Visual Stimuli Orientation and Direction Selectivity in a Robotic Context. Frontiers in Neurorobotics vol. 12, Article 75, 10 pages.
O
-
Orbán, L. L., Plowright CMS, Chartier, S., Thompson, E., & Xu, V. (2015) Visual Choice Behaviour by Bumblebees (Bombus impatiens) Confirms Unsupervised Neural Network’s Prediction. Journal of Comparative Psychology. Vol.129, No. 3, 229-236.
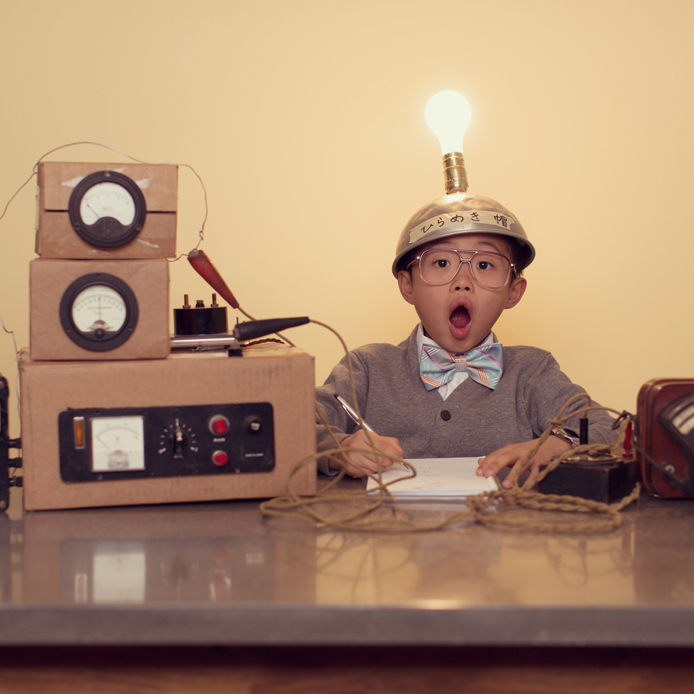
Le développement des architectures et les tâches non séparables linéairement
Habituellement les mémoires associatives bidirectionnelles (MAB) peuvent classifier seulement dans le cas où les données peuvent être séparées par une droite. Ce type d'apprentissage supervisé est courant dans la vie de tous les jours. Par exemple, faire l'association entre un nom et un numéro de téléphone. Ce type de classification est robuste au bruit et l'apprentissage peut être généralisé à de nouvelles données. En logique les porte OU et ET en sont des exemples. Cependant, il y plusieurs situations ou cette séparation linéaire n'est pas suffisante. Un exemple connu en psychologie est le problème de la balance où le participant doit intégrer l'information concomitante de deux dimensions: le poids et la distances. L'exemple classique en logique est celui du OU Exclusif. Les réseaux multicouches peuvent effectuer ce type de classification aisément. Malheureusement, la majorité d'entre eux manque de plausibilité cognitive ou biologique. Par conséquent, si nous voulons accroître la puissance explicative de la MAB, il est essentiel que cette dernière puisse résoudre les tâches non séparables linéairement (1). Ainsi, les apprentissages supervisés simples et complexes peuvent être incorporés au sein d'un modèle unique. Une solution possible serait d'utiliser une architecture croissante (ex. cascade correlation, (2)). Il fut montré que cette solution permet à des réseaux de résoudre ce type d'associations (3). Par conséquent, nous proposons qu'une fonction de croissance soit ajoutée au modèle.
- Chartier, S., Leth-Steensen, C. & Hébert, M.-F. (2012). Performing Complex Associations Using a Generalized Bidirectional Associative Memory. Journal of Experimental & Theoretical Artificial Intelligence, vol. 24, no. 1, pages 23-42
- Fahlman, S. E., & Lebiere, C. (1990). The cascade-correlation learning architecture. Advances in Neural Information Processing Systems II, 524-532.
- Tremblay, C., Myers-Stewart, K., Morissette, L., & Chartier, S. (2013, July). Bidirectional Associative Memory and Learning of Nonlinearly Separable Tasks. In R. West & T. Stewart (Eds.), Proceedings of the 12th International Conference on Cognitive Modeling, Ottawa, Canada, pp. 420-425.
L'apprentissage par renforcement dans les mémoires associatives
Les apprentissages supervisés et non supervisés sont de nature passive; les bonnes réponses sont données pour chaque valeur de sortie. Ceci contraste avec l’apprentissage par renforcement où le réseau se doit d’être actif. Il doit être en mesure de générer une action (réponse) à laquelle seulement une rétroaction de réussite ou échec est donnée. Ainsi, lorsque le réseau n’émet pas la bonne action, ce dernier doit pouvoir en essayer une autre solution potentielle basée sur ses acquis (exploitation). Dans certaines situations, le réseau peut avoir épuisé toutes les solutions possibles and doit par conséquent en générer une nouvelle (exploration) (1); . Étant donné que la MAB est de nature passive, peu d’essais ont été faits afin d’implémenter l’apprentissage par renforcement. Deux solutions furent tentées dans le passées. La première vise à contrôler des réponses et l’ajout d’une exploration chaotique (2). La deuxième utilise l’implémentation du Q-learning à l’intérieur de la règle d’apprentissage de la MAB (3). Dans les deux cas, les résultats ont montré la possibilité d'utiliser l’apprentissage par renforcement dans les réseaux récurrents.
- Sutton, R. S. & Barto, A. G. (1998). Reinforcement Learning: An Introduction. Cambridge: MIT Press.
- Chartier, S. Boukadoum, M. & Amiri, M. (2009). BAM learning of nonlinearly seperable tasks by using an asymmetrical output function and reinforcement learning, IEEE Transactions on neural networks, vol. 20, pp. 1281-1292.
- Salmon, R., Sadeghian, A. & Chartier, S. (2010). Reinforcement learning using associative memory networks, Proceedings of the International Joint Conference on Neural Networks, Barcelona, Spain, 7 pages.
La plasticité dans le développement des circuits neuronaux
Les changements dépendants de l’activité dans les transmissions synaptiques proviennent de grand nombre de mécanismes connus sous la plasticité synaptique. Cette dernière peut être divisée selon trois grandes catégories : (1) la plasticité à long terme, où les changements peuvent durer des heures ou plus permettant l’apprentissage et la mémoire; (2) la plasticité homéostatique, où les synapses et les neurones maintiennent l’excitabilité et la connectivité malgré des changements abruptes résultant la dépendance à l’expérience de la plasticité; (3) la plasticité à court-terme, où les changements dans la force des synapses se produisent dans l’ordre des millisecondes aux secondes. Dans les circuits neuronaux, les codes neuronaux pour les items chargés montrent des patrons d’activités nécessitant d’être distinguables des autres qui demeurent à un niveau d’activité de base. À l’intérieur de ce schème, cela demeure encore inconnu comment les contributions collectives des plasticités à court et long terme ainsi qu’homéostatique permettent le gain, le maintien ou la perte d’information dans les circuits neuronaux. Afin de s’attaquer à ce problème, il est important d’examiner l’interaction synergique entre ces mécanismes distincts et omniprésents de la plasticité dans les circuits neuronaux.
- Berberian N., Ross M., Chartier S., Thivierge J.P. (2017). Synergy Between Short-Term and Long-Term Plasticity Explains Direction Selectivity in Visual Cortex. IEEE Symposium Series on Computational Intelligence (IEEE SSCI), 1-8.
- Costa R P., Froemke R. C., Sjöström P. J., & van Rossum M. C. W. (2015). Unified pre- and postsynaptic long-term plasticity enables reliable and flexible learning, Elife, vol. 4, pp. 1–16.
- Mongillo G., Barak O., & Tsodyks M. (2008). Synaptic Theory of Working Memory. Science, vol. 319, no. 5869, pp. 1543–1546.
L'apprentissage des concepts de relation
L’apprentissage des concepts de relation représente un processus d’abstraction de règles entres les stimuli sans références précises quant à leurs caractéristiques physiques. Plusieurs espèces animales montrent cette capacité (1). Par exemple, le concept de relation de haut/bas peut être appris pas des agents naturels mêmes des invertébrés (2). Par ailleurs, il est possible que plus d’une relation puisse être apprises en concomitance (3). Même si les données empiriques provenant des organismes neuronaux simples sont connues, il n’en demeure pas moins qu’un circuit précis impliquant le processus d’apprentissage des concepts de relation reste à être trouvé. Ce phénomène cognitif peut aussi être étudié par différents outils computationnels, tels que les réseaux de neurones à impulsions contrôlant un robot (4). Un des objectifs du laboratoire est de mettre au défi nos modèles en tenant compte des faits de ce processus cognitif provenant d’une perspective d’intelligence artificielle.
- Zentall, T. R., Wasserman, E. A., & Urcuioli, P. J. (2014). Associative concept learning in animals. Journal of the experimental analysis of behavior, 101(1), 130-151.
- Avarguès-Weber, A., Dyer, A. G., & Giurfa, M. (2010). Conceptualization of above and below relationships by an insect. Proceedings of the Royal Society of London B: Biological Sciences, rspb20101891.
- Avarguès-Weber, A., Dyer, A. G., Combe, M., & Giurfa, M. (2012). Simultaneous mastering of two abstract concepts by the miniature brain of bees. Proceedings of the National Academy of Sciences, 201202576.
- Cyr, A., Avarguès-Weber, A., & Theriault, F. (2017). Sameness/difference spiking neural circuit as a relational concept precursor model: A bio-inspired robotic implementation. Biologically Inspired Cognitive Architectures, 21, 59-66.
Laboratoire de cognition et neurodynamique computationnelle
École de psychologie
Faculté des sciences sociales
Université d'Ottawa
136 Jean-Jacques Lussier
Pavillon Vanier, pièce 3022
Ottawa, Ontario, Canada K1N 6N5
Carte